Yes. We published this as open source, we contributed it to Apache, and, updating this answer yet again I'll add that we have pretty much moved a lot of our focus onto Storm and other technologies too. Note: We publish updates on our work with Storm and other technologies on our Tumblr here. http://yahoohadoop.tumblr.com/post/98751512631/the-evolution-of-storm-at-yahoo-and-apache
Previously Updated Answer: This project <strike>has been approved for being Open Sourced and will be</strike> is available on Github at http://github.com/s4 . You should see the S4 codebase available to you <strike>s
Yes. We published this as open source, we contributed it to Apache, and, updating this answer yet again I'll add that we have pretty much moved a lot of our focus onto Storm and other technologies too. Note: We publish updates on our work with Storm and other technologies on our Tumblr here. http://yahoohadoop.tumblr.com/post/98751512631/the-evolution-of-storm-at-yahoo-and-apache
Previously Updated Answer: This project <strike>has been approved for being Open Sourced and will be</strike> is available on Github at http://github.com/s4 . You should see the S4 codebase available to you <strike>soon</strike> now. (the code and website content is being staged by the team at http://s4.io/).
We are glad to learn that people are interested in this and we hope that you consider contributing bug fixes and the like to improve the code over time.
I hope this answer helps.
Another update:
S4 has been contributed to the Apache Software Foundation Incubator:
See http://incubator.apache.org/projects/s4.html and http://wiki.apache.org/incubator/S4Proposal
So going forward, look to the Apache process for updates to S4.
And I'll note that we don't call it "Real-Time MapReduce" -- we call it "Stream-processing" and we think this is clearer and more accurate.
Where do I start?
I’m a huge financial nerd, and have spent an embarrassing amount of time talking to people about their money habits.
Here are the biggest mistakes people are making and how to fix them:
Not having a separate high interest savings account
Having a separate account allows you to see the results of all your hard work and keep your money separate so you're less tempted to spend it.
Plus with rates above 5.00%, the interest you can earn compared to most banks really adds up.
Here is a list of the top savings accounts available today. Deposit $5 before moving on because this is one of th
Where do I start?
I’m a huge financial nerd, and have spent an embarrassing amount of time talking to people about their money habits.
Here are the biggest mistakes people are making and how to fix them:
Not having a separate high interest savings account
Having a separate account allows you to see the results of all your hard work and keep your money separate so you're less tempted to spend it.
Plus with rates above 5.00%, the interest you can earn compared to most banks really adds up.
Here is a list of the top savings accounts available today. Deposit $5 before moving on because this is one of the biggest mistakes and easiest ones to fix.
Overpaying on car insurance
You’ve heard it a million times before, but the average American family still overspends by $417/year on car insurance.
If you’ve been with the same insurer for years, chances are you are one of them.
Pull up Coverage.com, a free site that will compare prices for you, answer the questions on the page, and it will show you how much you could be saving.
That’s it. You’ll likely be saving a bunch of money. Here’s a link to give it a try.
Consistently being in debt
If you’ve got $10K+ in debt (credit cards…medical bills…anything really) you could use a debt relief program and potentially reduce by over 20%.
Here’s how to see if you qualify:
Head over to this Debt Relief comparison website here, then simply answer the questions to see if you qualify.
It’s as simple as that. You’ll likely end up paying less than you owed before and you could be debt free in as little as 2 years.
Missing out on free money to invest
It’s no secret that millionaires love investing, but for the rest of us, it can seem out of reach.
Times have changed. There are a number of investing platforms that will give you a bonus to open an account and get started. All you have to do is open the account and invest at least $25, and you could get up to $1000 in bonus.
Pretty sweet deal right? Here is a link to some of the best options.
Having bad credit
A low credit score can come back to bite you in so many ways in the future.
From that next rental application to getting approved for any type of loan or credit card, if you have a bad history with credit, the good news is you can fix it.
Head over to BankRate.com and answer a few questions to see if you qualify. It only takes a few minutes and could save you from a major upset down the line.
How to get started
Hope this helps! Here are the links to get started:
Have a separate savings account
Stop overpaying for car insurance
Finally get out of debt
Start investing with a free bonus
Fix your credit
Update: http://s4.io is live. The GitHub page is at https://github.com/s4/core
Looks like S4 is more of a stream processing system rather than mapreduce.
How does this compares with Google's Percolator?
Hi
- In fact, its 32,000-node cluster is still the largest in the world. Now the Web giant is souping up its massive investment in Hadoop to give it a deep learning environment that’s the envy of the valley. With more than 600 petabytes of data spread across 40,000 Hadoop nodes, Yahoo is a big believer in all things Hadoop.
Hi
- In fact, its 32,000-node cluster is still the largest in the world. Now the Web giant is souping up its massive investment in Hadoop to give it a deep learning environment that’s the envy of the valley. With more than 600 petabytes of data spread across 40,000 Hadoop nodes, Yahoo is a big believer in all things Hadoop.
Today (April 2, 2012)
Listen in to JSConf 2012 (or follow the tweetstream) http://2012.jsconf.us/#/schedule where you'll get an update about Mojito and the Open Source plans. We put the code on http://github.com/yahoo/mojito
Read it, fork it, play with it, test it out, enhance it, suggest enhancements, add to it, make great stuff with it, and let's take Node.JS to the next level, as a community.
Today (April 2, 2012)
Listen in to JSConf 2012 (or follow the tweetstream) http://2012.jsconf.us/#/schedule where you'll get an update about Mojito and the Open Source plans. We put the code on http://github.com/yahoo/mojito
Read it, fork it, play with it, test it out, enhance it, suggest enhancements, add to it, make great stuff with it, and let's take Node.JS to the next level, as a community.
There were about 36,000 nodes when I left in April 2010 and we were building out a new data center for Hadoop, in addition to the existing two data centers from 2009.
A dedicated IP offers several advantages for businesses needing a consistent, reliable online presence and secure remote access.
- Remote access enablement. For businesses with remote employees, a dedicated IP provides an opportunity to establish a remote connection to the company network from any location, allowing efficient remote work. NordLayer provides this through easy-to-use Virtual Private Gateways that are available in both monthly and yearly plans. Discover more here.
- Reliable website hosting and communication. If your business hosts its own website or email server, a static IP ensures r
A dedicated IP offers several advantages for businesses needing a consistent, reliable online presence and secure remote access.
- Remote access enablement. For businesses with remote employees, a dedicated IP provides an opportunity to establish a remote connection to the company network from any location, allowing efficient remote work. NordLayer provides this through easy-to-use Virtual Private Gateways that are available in both monthly and yearly plans. Discover more here.
- Reliable website hosting and communication. If your business hosts its own website or email server, a static IP ensures reliable connectivity, making it easier for customers to find your site and communicate with you. It also benefits voice-over-IP (VoIP) services, ensuring high-quality calls.
- Enhanced security. With a static IP, you can set up IP allowlisting to permit only greenlighted IP addresses to connect to your organization's network, service, or resource, which adds extra protection against unauthorized access. Explore IP allowlisting and other security features with NordLayer plans.
Overall, a dedicated IP enhances connectivity, supports reliable hosting, and boosts security. If that's what you're looking for, check out NordLayer's solutions. Enjoy a 22% discount on yearly plans and a 14-day money-back guarantee.
If you have been carefully following yahoo since 2009, you might be knowing that they have sold large part of business along with the brand yahoo to Oath(subsidiary of Verizon). So, Yahoo might not have the large cluster as they use to have.
There are other organizations like Facebook,Instagram and SnapChat which have a large user base and might have bigger cluster right now.
If you just want to write and run MapReduce jobs locally without a Hadoop cluster, you could consider the HackReduce starter kit. At one of the HackReduce events, I was able to use the configuration the organizers provided to quickly get started coding a MapReduce program in Java/Eclipse, testing locally and eventually deploying the program to run on the EC2 clusters that were provided.
Their source code is available on GitHub at https://github.com/hopper/HackReduce.
To run a job locally, you could follow one of the commands beginning with "java -classpath" in the middle of their README file. Un
If you just want to write and run MapReduce jobs locally without a Hadoop cluster, you could consider the HackReduce starter kit. At one of the HackReduce events, I was able to use the configuration the organizers provided to quickly get started coding a MapReduce program in Java/Eclipse, testing locally and eventually deploying the program to run on the EC2 clusters that were provided.
Their source code is available on GitHub at https://github.com/hopper/HackReduce.
To run a job locally, you could follow one of the commands beginning with "java -classpath" in the middle of their README file. Unfortunately, I don't think there's a way to run your jobs on an actual Hadoop cluster as they don't seem to provide an AMI for their EC2 setup, and these clusters are probably only made available at HackReduce events.
If you want to explore setting up your own Hadoop cluster, their resources page (http://www.hackreduce.org/hadoop-and-mapreduce/) has several links to MapReduce and Hadoop tutorials which you might find useful.
With today’s modern day tools there can be an overwhelming amount of tools to choose from to build your own website. It’s important to keep in mind these considerations when deciding on which is the right fit for you including ease of use, SEO controls, high performance hosting, flexible content management tools and scalability. Webflow allows you to build with the power of code — without writing any.
You can take control of HTML5, CSS3, and JavaScript in a completely visual canvas — and let Webflow translate your design into clean, semantic code that’s ready to publish to the web, or hand off
With today’s modern day tools there can be an overwhelming amount of tools to choose from to build your own website. It’s important to keep in mind these considerations when deciding on which is the right fit for you including ease of use, SEO controls, high performance hosting, flexible content management tools and scalability. Webflow allows you to build with the power of code — without writing any.
You can take control of HTML5, CSS3, and JavaScript in a completely visual canvas — and let Webflow translate your design into clean, semantic code that’s ready to publish to the web, or hand off to developers.
If you prefer more customization you can also expand the power of Webflow by adding custom code on the page, in the <head>, or before the </head> of any page.
Trusted by over 60,000+ freelancers and agencies, explore Webflow features including:
- Designer: The power of CSS, HTML, and Javascript in a visual canvas.
- CMS: Define your own content structure, and design with real data.
- Interactions: Build websites interactions and animations visually.
- SEO: Optimize your website with controls, hosting and flexible tools.
- Hosting: Set up lightning-fast managed hosting in just a few clicks.
- Grid: Build smart, responsive, CSS grid-powered layouts in Webflow visually.
Discover why our global customers love and use Webflow | Create a custom website.
I can see many reasons:
- Simplicity - The simplicity makes it incredibly easy to understand.
- Applicability - The paradigm is so simple that a very large number of already defined algorithms can be implemented using one or more Map-Reduce steps. This makes it very easy to implement large scale distributed systems for things that required a lot more information regarding distributed systems than before.
- Scalability - The abstraction is implicitly scalable which takes makes it very useful for cases where the amount of data processing is huge.
- Redundancy - Error correction can be implemented pretty e
I can see many reasons:
- Simplicity - The simplicity makes it incredibly easy to understand.
- Applicability - The paradigm is so simple that a very large number of already defined algorithms can be implemented using one or more Map-Reduce steps. This makes it very easy to implement large scale distributed systems for things that required a lot more information regarding distributed systems than before.
- Scalability - The abstraction is implicitly scalable which takes makes it very useful for cases where the amount of data processing is huge.
- Redundancy - Error correction can be implemented pretty easily using redundancy. Many other paradigms find it hard to implement fault tolerance without significant work, Map-Reduce makes it easier. Most Map-Reduce deployments are on Distributed Filesystems (HDFS/BigTable), making the data retrieval/deposit steps fault tolerant as well.
- Infrastructure Requirements - Are not high. The underlying infrastructure is generic; a network of simple processing units with disk access.
No, MapReduce is not currently exposed on AppEngine. Some creative users have implemented "Map" on top of app engine here: http://code.google.com/p/appengine-mapreduce/ but they haven't gotten to the "reduce" side yet, apparently.
I once met a man who drove a modest Toyota Corolla, wore beat-up sneakers, and looked like he’d lived the same way for decades. But what really caught my attention was when he casually mentioned he was retired at 45 with more money than he could ever spend. I couldn’t help but ask, “How did you do it?”
He smiled and said, “The secret to saving money is knowing where to look for the waste—and car insurance is one of the easiest places to start.”
He then walked me through a few strategies that I’d never thought of before. Here’s what I learned:
1. Make insurance companies fight for your business
Mos
I once met a man who drove a modest Toyota Corolla, wore beat-up sneakers, and looked like he’d lived the same way for decades. But what really caught my attention was when he casually mentioned he was retired at 45 with more money than he could ever spend. I couldn’t help but ask, “How did you do it?”
He smiled and said, “The secret to saving money is knowing where to look for the waste—and car insurance is one of the easiest places to start.”
He then walked me through a few strategies that I’d never thought of before. Here’s what I learned:
1. Make insurance companies fight for your business
Most people just stick with the same insurer year after year, but that’s what the companies are counting on. This guy used tools like Coverage.com to compare rates every time his policy came up for renewal. It only took him a few minutes, and he said he’d saved hundreds each year by letting insurers compete for his business.
Click here to try Coverage.com and see how much you could save today.
2. Take advantage of safe driver programs
He mentioned that some companies reward good drivers with significant discounts. By signing up for a program that tracked his driving habits for just a month, he qualified for a lower rate. “It’s like a test where you already know the answers,” he joked.
You can find a list of insurance companies offering safe driver discounts here and start saving on your next policy.
3. Bundle your policies
He bundled his auto insurance with his home insurance and saved big. “Most companies will give you a discount if you combine your policies with them. It’s easy money,” he explained. If you haven’t bundled yet, ask your insurer what discounts they offer—or look for new ones that do.
4. Drop coverage you don’t need
He also emphasized reassessing coverage every year. If your car isn’t worth much anymore, it might be time to drop collision or comprehensive coverage. “You shouldn’t be paying more to insure the car than it’s worth,” he said.
5. Look for hidden fees or overpriced add-ons
One of his final tips was to avoid extras like roadside assistance, which can often be purchased elsewhere for less. “It’s those little fees you don’t think about that add up,” he warned.
The Secret? Stop Overpaying
The real “secret” isn’t about cutting corners—it’s about being proactive. Car insurance companies are counting on you to stay complacent, but with tools like Coverage.com and a little effort, you can make sure you’re only paying for what you need—and saving hundreds in the process.
If you’re ready to start saving, take a moment to:
- Compare rates now on Coverage.com
- Check if you qualify for safe driver discounts
- Reevaluate your coverage today
Saving money on auto insurance doesn’t have to be complicated—you just have to know where to look. If you'd like to support my work, feel free to use the links in this post—they help me continue creating valuable content.
Distributed sorting is a great benchmark for mapreduce implementations because it exercises all the parts of the framework well.
The most time consuming part probably is sending the data over the network from the mappers to the right reducers.
Over time a number of results on Distributed Sort benchmarks have been reported by Yahoo, Google and Microsoft:
- yahoo08 TeraByte Sort on Apache Hadoop http://sortbenchmark.org/YahooHadoop.pdf
- google08 Sorting 1PB with MapReduce http://googleblog.blogspot.com/2008/11/sorting-1pb-with-mapreduce.html
- yahoo09 Winning a 60 Second Dash with a Yellow Elephant http:
Distributed sorting is a great benchmark for mapreduce implementations because it exercises all the parts of the framework well.
The most time consuming part probably is sending the data over the network from the mappers to the right reducers.
Over time a number of results on Distributed Sort benchmarks have been reported by Yahoo, Google and Microsoft:
- yahoo08 TeraByte Sort on Apache Hadoop http://sortbenchmark.org/YahooHadoop.pdf
- google08 Sorting 1PB with MapReduce http://googleblog.blogspot.com/2008/11/sorting-1pb-with-mapreduce.html
- yahoo09 Winning a 60 Second Dash with a Yellow Elephant http://sortbenchmark.org/Yahoo2009.pdf
- google11 Sorting Petabytes with MapReduce - The Next Episode http://googleresearch.blogspot.com/2011/09/sorting-petabytes-with-mapreduce-next.html
- microsoft12 Data in the Fast Lane http://research.microsoft.com/en-us/news/features/minutesort-052112.aspx
Each benchmark was sorting through keys of 100 bytes in length.
Here's is a summary of the results (hope I didn't mess this up :) ):
cores was only reported in the Yahoo results and it was 8 cores per node.
I computed throughput as the average of how much data is sorted by a CPU per second.
The MinuteSort result is interesting because it has the highest throughput per machine. The purpose of the benchmark is sort as much data as possible in 60 seconds, so I wonder if using just 250 machines is a limitation of their system.
As for hadoop vs google map reduce in 2011, there's no new benchmark from Yahoo.
It's impressive how Google MR has improved between 2008 and 2011. Can use double the number of nodes, sort through 10 times the data and have a 5 times higher node throughput.
The google11 article mentions:
We are excited by these results. While internal improvements to the MapReduce framework contributed significantly, a large part of the credit goes to numerous advances in Google's hardware, cluster management system, and storage stack.
The list of people who worked on and/or directly influenced Hadoop as part of their official duties at Yahoo! pre-2009 is around 40 or so people. A lot of them have spread out to other companies/projects. Many of them are no longer directly involved with Hadoop. The best way to find out if someone was actually, directly involved is to ask which org they were in. If it wasn't specifically Grid, then they were likely (but not 100% guaranteed) just a user.
(or if they remember how many bugs we hit when we upgraded Mithril to support users and permissions in HDFS. haha.)
BTW, Mike was never an em
The list of people who worked on and/or directly influenced Hadoop as part of their official duties at Yahoo! pre-2009 is around 40 or so people. A lot of them have spread out to other companies/projects. Many of them are no longer directly involved with Hadoop. The best way to find out if someone was actually, directly involved is to ask which org they were in. If it wasn't specifically Grid, then they were likely (but not 100% guaranteed) just a user.
(or if they remember how many bugs we hit when we upgraded Mithril to support users and permissions in HDFS. haha.)
BTW, Mike was never an employee of Yahoo!. Like him, there are a handful of people at that time that were working on Hadoop outside of Y!. They are rare, but they exist. ;)
Flume is comparable; see How does S4 compare to Flume? for the differences.
At the most recent HBase hackathon, one idea we discussed doing in 2011 is something that would allow HBase to very closely approximate Haystack in a lot of ways.
The general idea is the same as what databases have been doing for a long time as external blob storage. When any row is written with a larger amount of data, we would append it to a "haystack" and then just write the metadata into the table itself. On retrieval, the metadata would be small enough to fit in cache and we would perform a single seek into the haystack to read the data.
The advantages here are:
- the large data wouldn't e
At the most recent HBase hackathon, one idea we discussed doing in 2011 is something that would allow HBase to very closely approximate Haystack in a lot of ways.
The general idea is the same as what databases have been doing for a long time as external blob storage. When any row is written with a larger amount of data, we would append it to a "haystack" and then just write the metadata into the table itself. On retrieval, the metadata would be small enough to fit in cache and we would perform a single seek into the haystack to read the data.
The advantages here are:
- the large data wouldn't ever have to hit the HLog, and hence would increase throughput
- the large data wouldn't have to be compacted (or could be compacted much more infrequently) while the metadata could continue to be compacted in order to provide efficient lookup
- the haystack files would be immutable over a long period, allowing HDFS improvements like HDFS RAID to reduce storage requirements.
- Ceph
- QFS by Quantcast: http://quantcast.github.com/qfs/
- Cloud-Crowd by DocumentCloud: https://github.com/documentcloud/cloud-crowd
- HPCC by LexisNexis: http://hpccsystems.com/
- Condor: http://www.cs.wisc.edu/condor/ (application notes: www.cse.nd.edu/~ccl/research/pubs/pbragahe-thesis.pdf )
- Storm by Nathan Marz: https://github.com/nathanmarz/storm
- HaLoop: An offshoot of Hadoop to support iterative data processing: http://code.google.com/p/haloop/
- MapRejuice: the distributed client-side computing implementation of Map Reduce built on top of Node.js: https://github.com/ryanmcgrath/maprejuice
- GoCircuit:
- Ceph
- QFS by Quantcast: http://quantcast.github.com/qfs/
- Cloud-Crowd by DocumentCloud: https://github.com/documentcloud/cloud-crowd
- HPCC by LexisNexis: http://hpccsystems.com/
- Condor: http://www.cs.wisc.edu/condor/ (application notes: www.cse.nd.edu/~ccl/research/pubs/pbragahe-thesis.pdf )
- Storm by Nathan Marz: https://github.com/nathanmarz/storm
- HaLoop: An offshoot of Hadoop to support iterative data processing: http://code.google.com/p/haloop/
- MapRejuice: the distributed client-side computing implementation of Map Reduce built on top of Node.js: https://github.com/ryanmcgrath/maprejuice
- GoCircuit: Paradigm for developing and sustaining Big Data apps
Subsequent to this question and two answers being provided, Yahoo! has indeed "spun off" a significant part of the Hadoop engineering team, forming the "Hortonworks" company http://www.hortonworks.com/ -- dedicating to delivering success with Hadoop. Yahoo! continues its significant investment in Hadoop engineering internally as this technology is very important to the company.
This is viewed as a "win-win" situation which should greatly benefit the Apache Hadoop community. It will help address the huge demand (and short supply) for expertise and services in this very sophisticated area of t
Subsequent to this question and two answers being provided, Yahoo! has indeed "spun off" a significant part of the Hadoop engineering team, forming the "Hortonworks" company http://www.hortonworks.com/ -- dedicating to delivering success with Hadoop. Yahoo! continues its significant investment in Hadoop engineering internally as this technology is very important to the company.
This is viewed as a "win-win" situation which should greatly benefit the Apache Hadoop community. It will help address the huge demand (and short supply) for expertise and services in this very sophisticated area of technology. And it provides a nice influx into the economics of Hadoop for Yahoo! and other ecosystem participants.
Hadoop consists of many subprojects: HDFS, MapReduce, Hive, Pig, HBase, and Avro. I believe this question refers to the MapReduce implementation, which can operate over a variety of storage systems. I agree that Hadoop could use decent competition; having used the software daily for many years, I disagree that it's becoming more complex with each release. As an example, see the heroic efforts of Chris Douglas on https://issues.apache.org/jira/browse/MAPREDUCE-64 to remove some of the tuning parameters for a MapReduce job. I suppose that's for another question though.
Both MapReduce implementati
Hadoop consists of many subprojects: HDFS, MapReduce, Hive, Pig, HBase, and Avro. I believe this question refers to the MapReduce implementation, which can operate over a variety of storage systems. I agree that Hadoop could use decent competition; having used the software daily for many years, I disagree that it's becoming more complex with each release. As an example, see the heroic efforts of Chris Douglas on https://issues.apache.org/jira/browse/MAPREDUCE-64 to remove some of the tuning parameters for a MapReduce job. I suppose that's for another question though.
Both MapReduce implementations mentioned above (CouchDB and MongoDB) require that data live in the data stores specified and present far different semantics than those described in the Google paper.
Here are some MapReduce-ish implementations, all of which are either coupled to a single storage system, a single programming language, or implement only a small subset of the features of a mature MapReduce implementation:
- Disco: http://discoproject.org
- Misco: http://www.cs.ucr.edu/~jdou/misco/
- Phoenix: http://mapreduce.stanford.edu
- Cloud MapReduce: http://code.google.com/p/cloudmapreduce
- bashreduce: http://blog.last.fm/2009/04/06/mapreduce-bash-script
- Qizmt: http://code.google.com/p/qizmt
- HTTPMR: http://code.google.com/p/httpmr
- Galago's TupleFlow: http://www.galagosearch.org/guide.html
- Skynet: http://skynet.rubyforge.org
- Sphere: http://sector.sourceforge.net
- Riak: http://riak.basho.com/mapreduce.html
- Starfish: http://rufy.com/starfish/doc/
- Octopy: http://code.google.com/p/octopy/
- MPI-MR: http://www.sandia.gov/~sjplimp/mapreduce.html
- Filemap: http://mfisk.github.com/filemap/
- Plasma MapReduce: http://projects.camlcity.org/projects/plasma.html
- Mapredus: http://rubygems.org/gems/mapredus
- Mincemeat: http://remembersaurus.com/mincemeatpy/
- MapReduceTitan: http://www.kitware.com/InfovisWiki/index.php/MapReduce
- GPMR: http://www.idav.ucdavis.edu/research/projects/mgpu_mapreduce
- Elastic Phoenix: https://github.com/adamwg/elastic-phoenix
- Preregrine: http://peregrine_mapreduce.bitbucket.org/
- R3: http://heynemann.github.com/r3/
Also, Microsoft's DryadLINQ is available under an academic license (not quite open source) at http://research.microsoft.com/en-us/projects/Dryad.
Disclosure: I founded Cloudera, a company that provides commercial support for a distribution of Hadoop, among other things.
The pipe2py open-source project runs Yahoo! Pipes locally using Python (https://github.com/ggaughan/pipe2py). This can then be run on Google App Engine for scalability (http://www.wordloosed.com/running-yahoo-pipes-on-google-app-engine).
I believe the answer is "No". Contrary to some answers, the superiority of MPP systems for analyzing relational data has little to do with "ACID compliance"--indeed, many of these systems are used entirely offline, where consistency concerns are irrelevant. They are faster than Hadoop because they are optimized for relational operations, and because they don't make some of the dubious design decisions (such as materializing all intermediate results for fault tolerance) that appeared in the original MapReduce paper. Even later Hadoop iterations (e.g., Hive on Tez) don't perform as well on relat
I believe the answer is "No". Contrary to some answers, the superiority of MPP systems for analyzing relational data has little to do with "ACID compliance"--indeed, many of these systems are used entirely offline, where consistency concerns are irrelevant. They are faster than Hadoop because they are optimized for relational operations, and because they don't make some of the dubious design decisions (such as materializing all intermediate results for fault tolerance) that appeared in the original MapReduce paper. Even later Hadoop iterations (e.g., Hive on Tez) don't perform as well on relational queries as purpose-built MPP systems like Impala or Presto.
Shameless plug: the University of Washington database group is working on such an MPP system that is compatible with HDFS: http://myria.cs.washington.edu
In short, yes you can do MR on AppEngine. Here are some links:
- AppEngine-MapReduce: http://code.google.com/p/appengine-mapreduce/
- Success Story: http://www.google.com/buzz/bslatkin/6SXDRWXFWkN
- Google I/O 2010 Video: http://www.youtube.com/watch?v=_7fJotosrNQ
Bing uses a file system called Cosmos [1] that is conceptually similar to HDFS. Dryad [2] is their execution infrastructure; it's more expressive than MapReduce. The majority of the queries run over Cosmos are done with SCOPE [3], which is conceptually similar to Hive.
[1] http://www.goland.org/whatiscosmos
[2] http://dl.acm.org/citation.cfm?id=1273005
[3] http://dl.acm.org/citation.cfm?id=1454166
No. At this time it is not on a roadmap to be open sourced. Individuals who were working on the project to open source Sherpa have left the company. One component, Apache Traffic Server did get open sourced. Sherpa is unlikely at this point to be open sourced.
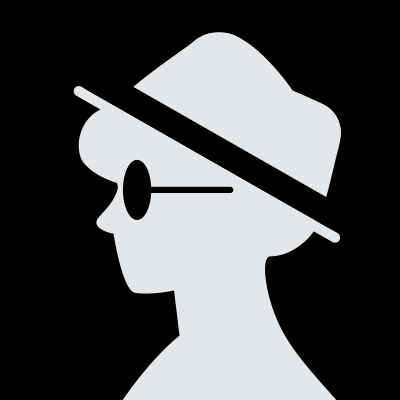
See, databases and mapreduce are entirely two different things. Mapreduce systems are intended for offline processing where as the databases/parallel databases are meant for online transaction processing. If we go with the published results mapreduce obviously beats the other warehouse analytics at large scale computation ("big data" they call it now). However if you worry about the scaling online transaction processing systems like databases and parallel databases, they pose an entirely new set of problems related to consistency and stuff (you can look into CAP theorem). Though I agree with y
See, databases and mapreduce are entirely two different things. Mapreduce systems are intended for offline processing where as the databases/parallel databases are meant for online transaction processing. If we go with the published results mapreduce obviously beats the other warehouse analytics at large scale computation ("big data" they call it now). However if you worry about the scaling online transaction processing systems like databases and parallel databases, they pose an entirely new set of problems related to consistency and stuff (you can look into CAP theorem). Though I agree with you that there has been no popular parallel database till date, most of it is because of the overhead they incur because they stick to ACID properties. But recent research and use-cases have proved that one need not stick to all of the ACID properties and some of them might be relaxed. This resulted in the new key-value stores where they address issues by trading some ACID properties (for eg: relaxing consistency rules) for performance and this has shown some improvement. You can look into systems like HBase, Cassandra, Google F1 database and read some literature on CAP theorem, eventual consistency etc. Overall I feel that there has been no big parallel database because they tried to stick to ACID properties thus giving a large overhead. However modern systems are trying to bridge that gap by relaxing something or the other that they feel is unnecessary. This is my opinion and comments are welcome.
If your main criteria is simplicity, I think that the two main alternatives are Disco (http://discoproject.org/) and Filemap (http://mfisk.github.com/filemap), both mentioned in a previous answer. I particularly like Filemap because you can't get mapreduce like processing simpler that this.
One interesting alternative is cloud mapreduce (http://code.google.com/p/cloudmapreduce/) which is implemented on top of Amazon Web Services and follows a completely different architecture without a job tracker, on which individual nodes self-schedulle the task of a job.
I don't agree with some (most) answ
If your main criteria is simplicity, I think that the two main alternatives are Disco (http://discoproject.org/) and Filemap (http://mfisk.github.com/filemap), both mentioned in a previous answer. I particularly like Filemap because you can't get mapreduce like processing simpler that this.
One interesting alternative is cloud mapreduce (http://code.google.com/p/cloudmapreduce/) which is implemented on top of Amazon Web Services and follows a completely different architecture without a job tracker, on which individual nodes self-schedulle the task of a job.
I don't agree with some (most) answers that mention nosql databases that include a form of map reduce, as those are not generic solutions: you must use that particular db in order to use their implementation of map reduce.
there's a notable exception: riak (http://riak.basho.com). Even when its implementation of map reduce is in principle tied to the db, in reality its architecture as evolved towards a more generic sustrate for distributed applications and the implementation of map reduce is much more generic. I don't believe it's still a fully independent mapreduce engine, but I would bet it's close (I've consider working on that direction myself)
With respect of some framework like phoenix and mars, they don't qualify as complete alternatives to hadoop as are targeted to multicore and/or graphic processor architectures and don't consider the distribution over large clusters.
Finally, I would consider storm (https://github.com/nathanmarz/storm). Even when it doesn't implement a map reduce model, its stream processing capabilities offer an alternative programming model and make relatively easy to implement map-reduce-like jobs.
Apache Spark is used. So is Apache Storm. So is Apache Hadoop. So are many related technology projects -- used in different parts of the company for different things. It's not about switching gears, it's about using the best tools for the job. There are many different products and platforms -- and they have differing use cases. So you'd expect Yahoo to rely upon many elements of the Big Data ecosystem, and not interpret this as a move from one to the other. Moreover, it's important for the large company to develop competence in many (seemingly competing) technologies so that they can expand op
Apache Spark is used. So is Apache Storm. So is Apache Hadoop. So are many related technology projects -- used in different parts of the company for different things. It's not about switching gears, it's about using the best tools for the job. There are many different products and platforms -- and they have differing use cases. So you'd expect Yahoo to rely upon many elements of the Big Data ecosystem, and not interpret this as a move from one to the other. Moreover, it's important for the large company to develop competence in many (seemingly competing) technologies so that they can expand options in case some workloads are better applied to one compute style vs another.
Come to the http://2015.hadoopsummit.org/ to learn more.
Yes.
Yahoo recently released an updated product report for 2015, highlighting the closure of several products, regional sites, and support for older Apple devices in some cases.
Maps is among the products that will be closed by the end of June. But the other Yahoo products will continue to support maps.
This is the snippet from the full report:
The Yahoo Maps site will close at the end of June. However, in the context of Yahoo search and on several other Yahoo properties including Flickr, we will continue to support maps. We made this decision to better align resources to Yahoo’s priorities as o
Yes.
Yahoo recently released an updated product report for 2015, highlighting the closure of several products, regional sites, and support for older Apple devices in some cases.
Maps is among the products that will be closed by the end of June. But the other Yahoo products will continue to support maps.
This is the snippet from the full report:
The Yahoo Maps site will close at the end of June. However, in the context of Yahoo search and on several other Yahoo properties including Flickr, we will continue to support maps. We made this decision to better align resources to Yahoo’s priorities as our business has evolved since we first launched Yahoo Maps eight years ago
If they have some unique IP, tools, etc. it could make sense. With the acquisition of Aster Data, Greenplum, Vertica, and Netezza the broader market obviously sees the value of coming up with better ways to deal with "big data". If Yahoo! engineers have developed a uniquely compelling way to do use Hadoop it then it would likely be a solid move.
I think the difference in in-memory processing. There are many ways to achieve it but it's pretty clear that batch-oriented MR is fading (albeit slowly). Google, facebook, twitter, etc. have realized that real time processing can't be accomplished w/o in-memory processing - and move on.
Yes, and (almost certainly) yes.
MapReduce has been modified and improved since the paper describing it was published, but it is certainly still in use. You can even use it yourself as part of Google App Engine (MapReduce Overview).
As for having something new, Google always has something new that they're not ready to publish; they publish hundreds of papers a year, Research at Google, many on algorithms, data structures, and distributed systems. I doubt they are working on a direct replacement for MapReduce, but they're frequently making new products incorporating MapReduce.
Google (company)'s relationship with Hadoop has always been sort of interesting.
In the early days, Google (along with IBM (company)) built some educational training materials for universities to use for teaching MapReduce to its students [1]. These materials utilized Hadoop because Google's internal secret sauce was still pretty much hidden away.
Additionally, there was some work done to add users to Hadoop with funding by Google.
After Christophe Bisciglia left Google, their direct contributions have mostly dried up. But they still remain a 'force' in the ecosystem if only for Google Summer o
Google (company)'s relationship with Hadoop has always been sort of interesting.
In the early days, Google (along with IBM (company)) built some educational training materials for universities to use for teaching MapReduce to its students [1]. These materials utilized Hadoop because Google's internal secret sauce was still pretty much hidden away.
Additionally, there was some work done to add users to Hadoop with funding by Google.
After Christophe Bisciglia left Google, their direct contributions have mostly dried up. But they still remain a 'force' in the ecosystem if only for Google Summer of Code (GSoC) and the occasional paper.
It's worth noting that Google has publically stated that they will not pursue Hadoop for any MapReduce patent violations. [2]
1 - http://googlepress.blogspot.com/2007/10/google-and-ibm-announce-university_08.html
2 - http://gigaom.com/2010/01/19/why-hadoop-users-shouldnt-fear-googles-new-mapreduce-patent/ amongst others.
MapReduce is not a database. It's a programming model that let's you split a task in a set of smaller jobs that can run in parallel and whose results can be fed recursively to another task and so forth. It's a mechanism to process lots of data in parallel.
There are databases that support running MapReduce jobs on the data that's stored in a cluster, and as such you can see them as complementary technologies.
Yahoo! has stopped updating the YDN Hadoop blog because they fully committed to Apache Hadoop (i.e. they stopped maintaining their own separate fork of Hadoop). In 2011, the folks working on Hadoop within Yahoo! spun off to found Hortonworks.